According to the World Health Organization (WHO), lung cancer is the deadliest type of cancer worldwide, accounting for 1.8 million deaths in 2020. The best way to treat cancer is early diagnosis, with the most common diagnostic procedures being chest radiography, radiography, and magnetic resonance imaging (MRI). Computed tomography (CT), on the other hand, is considered to be one of the most accurate diagnostic tools, which allows medical specialists to examine tissues layer by layer and detect tumors at early stages.
The diagnostic process goes as follows: a radiologist interprets an image of a patient’s lungs, scans it for tumors (if detected, they need to check if it is a benign or malignant one), and recommend treatment or additional testing when appropriate.
To obtain the results of their CT testing, patients can sign up for an in-person appointment or take an online consultation through telehealth services, like Yandex.Health, SberMedAI, and others, which are especially popular among those who want to receive a second medical opinion or struggle to get an appointment with their doctor.
If a patient wants to have an online consultation, they need to upload their CT scan for the doctor to examine. After they have examined the image, the doctor will inform the patient of the results by phone or forward them a medical conclusion.
Nevertheless, computer vision can be an invaluable asset for diagnostics automation. This is a technology that can automatically obtain, process, and describe images to solve an array of applied tasks. It is commonly used in production, as well as security and vision systems. It is also being actively introduced in medical practice, with half of Moscow’s clinics using AI in radiotherapy, according to the Moscow City Health Department.
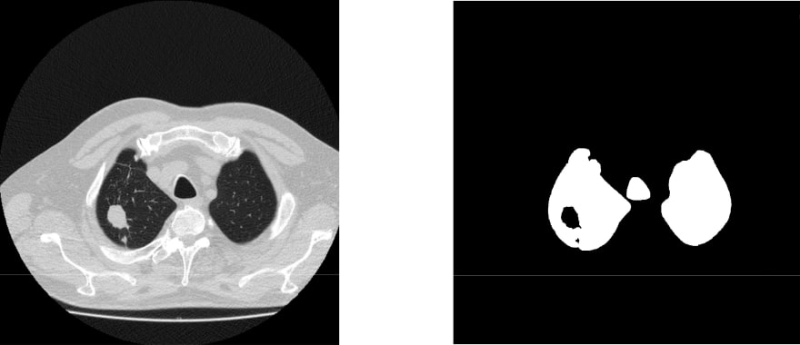
A CT scan of lung cancer: before watershed segmentation (left) and afterward (right). Illustrations by the project’s authors
ITMO researchers, too, work on health-related AI applications. In 2020, a team of the university’s scientists proposed an algorithm that can analyze an image in 2.15 seconds, with its accuracy hovering over 99%. The results are confirmed with the F1 score, i.e. an evaluation metric that measures a model's accuracy. The model demonstrated an F1 score of 0.996 (out of 1). As a general rule of thumb, the higher the score, the better the performance.
The solution consecutively applies two models: while the first one highlights lung cavities in an image, the other looks for any abnormal areas in the lungs using a neural network-based classifier. A two-stage analysis causes the system to run slowly – and therefore process fewer images – which makes it inapplicable in telehealth services.
A new solution
To accelerate the diagnostic process, students and staff of ITMO’s Higher School of Digital Culture decided to modify the earlier-proposed model and produced an improved system that delivers the same result over two times faster (0.38 vs 2.15 seconds).
The researchers replaced the detector with a novel solution, namely, a custom U-Net (a convolutional neural network for biomedical image segmentation).
The model was trained and tested on over 10,000 CT scans from two open-source databases: Moscow Radiology and The Cancer Imaging Archive.The conducted experiments proved that the solution retained the same level of accuracy at over 99% (the F1 score being 0.996).
What’s next
According to the developers, the algorithm can be integrated into online medical services, without any intent to replace human specialists but rather to serve as an assistant that can offer a second opinion.
“One of the major trends in computer vision is automation of clinical practices and examinations in particular. The integration process is complex and time-demanding, that's why it's so vital for researchers to continue developing the field. We decided to focus on online consultations,” shared Alexander Saveliev, a member of the team and a student at ITMO’s Higher School of Digital Culture.
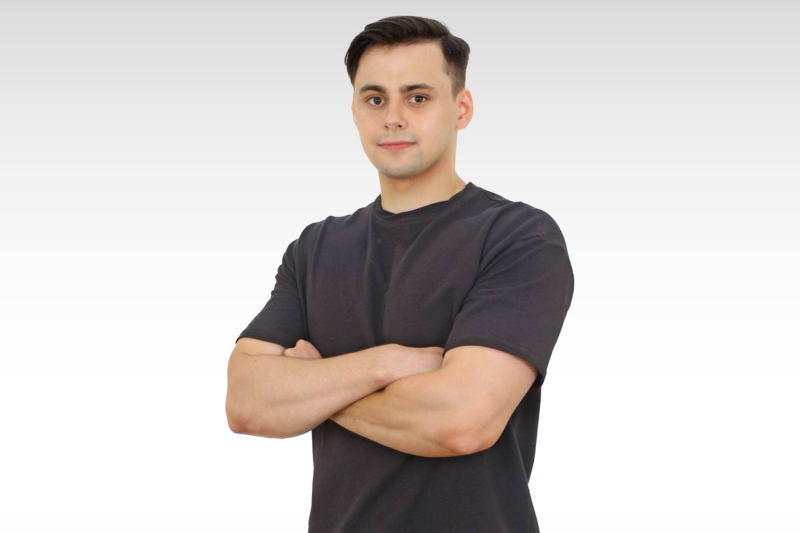
Alexander Saveliev. Photo courtesy of the subject
The project was prepared for ITMO’s grant contest for Master’s and PhD students. Within the contest, participants run studies on real-life data, apply for grants, write scientific papers, and present their projects at conferences. Learn more about the contest and apply here (in Russian).